Big data is one of the top technology trends for middle market companies. With it comes data modeling, or the manipulation and analysis of information to help better understand how a business can and should work. Smart use of the technology and methods can help a business get more competitive and hit the next stage of growth.
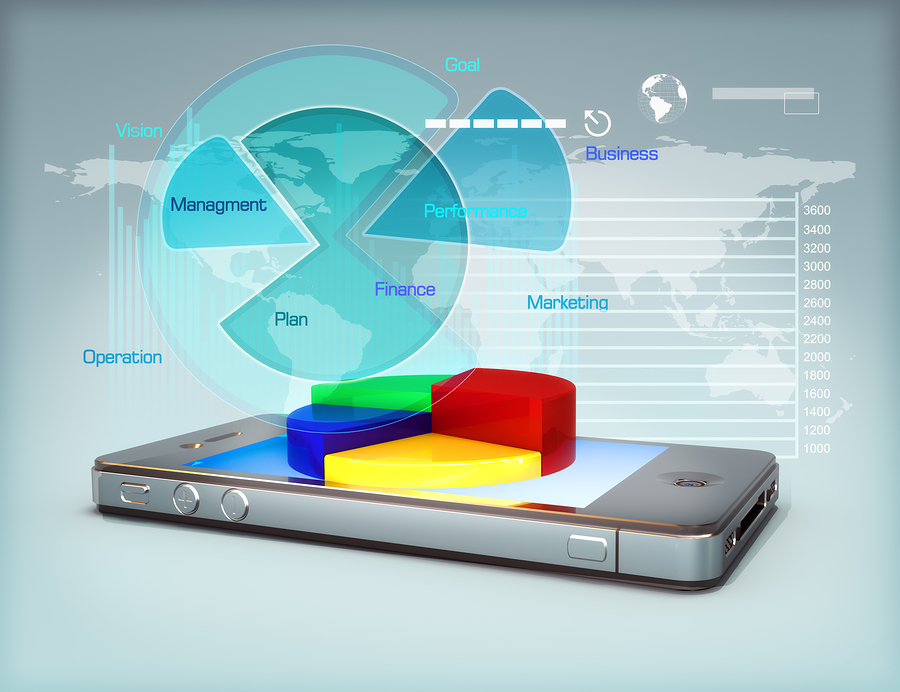
Data-driven decision processes left to their own and then unquestioned, however, can offer as many problems as benefits. When things are done badly, you're better off ignoring the data. Here's when you should at the very least be skeptical of the results you get.
When the Data Is Bad
The results of data modeling depend on the quality of data used. If there are errors in the collected information, formatting issues, inconsistent definitions of what data mean, or other such quality issues, you could get something that sends you off in the wrong direction. And then there are more subtle things that can go wrong, like failing to use enough variables to give a good picture of what is happening. It's like the old computer saying: "Garbage in, garbage out."
When the Problem You Solved Isn't the Problem You Have
Something else that can easily go wrong in data modeling is defining the problem you have. Actually, make that the problem you think you have. People in general, and executives are no exception, will often focus on one aspect of a situation and overlook other significant ones. I can remember one company that had an inbound sales force. The company wanted the salespeople to spend more time on the phone with customers to upsell and cross-sell. Each salesperson, however, was compensated in part on how quickly they could get off the phone to make room for more inbound calls. So, nothing changed because the data management looked at didn't square with the problem of paying people to get to the next call.
When You Reverse-Engineer Data to Support an Existing Theory
Research in cognitive science shows people form mental frameworks about virtually everything. People then interpret new data to fit the existing framework. They are re-engineering the information to support the theory they want to believe. A classic example is the medical community's treatment of stomach ulcers. For many years practitioners assumed that stress and excess acid caused them. In reality, the cause was bacteria, easily treated with antibiotics, that happened to sometimes increase acidity. It wasn't until the 1980s that a researcher swallowed some of the bacteria and developed ulcers and then wrote about the experience in a paper that the medical establishment finally believed the truth. Don't assume the solution and then look for justification, otherwise you waste time and money pulling together and analyzing the data.
When the Results Aren't Significant
In surveys there is the concept of the margin of error. Results are absolute numbers, but ranges you would get if you repeated the survey enough times drawing people from the same population. But come some political campaign, inevitably a reporter will talk about one candidate being ahead of the other, even if the gap is smaller than the margin of error. In that case, the positions of the candidates could just as easily be reversed. That is one situation in which results might not be significant. There are others, as well, like being told you could improve sales by a small percent but at a cost that would more than obliterate the additional gross margin.
When the Modelers Are Winging It
Data modeling and analysis tools are often so powerful and apparently near sentience that you'd think anyone could use them and get the right results. Only, that is never the case unless by accident. To see the potential problems, open a spreadsheet and look at the many statistical tools available, including ways of projecting future results. Depending on the technique you pick, the projection may be helpful or completely inaccurate. Data science requires significant judgment and not just the possession of the tools.
When You Don't Review the Data With the People Who Know It
Not involving the people who understand the data from the start of a modeling project is a good way to sink your efforts. There are too many cases where the modeling experts will misinterpret terminology, the importance of certain factors, or the relationships among different pieces of data.
Data modeling is a powerful tool, but not one to use lightly or casually. Be sure to have the expertise, tools, communication, people, and resources necessary to make your project work.
How does your company manage and implement data models?
Erik Sherman is an NCMM contributor and author whose work has appeared in such publications as The Wall Street Journal, The New York Times Magazine, Newsweek, the Financial Times, Chief Executive, Inc., and Fortune. He also blogs for CBS MoneyWatch. Sherman has extensive experience in corporate communications consulting and is the author or co-author of 10 books. Follow him on Twitter.